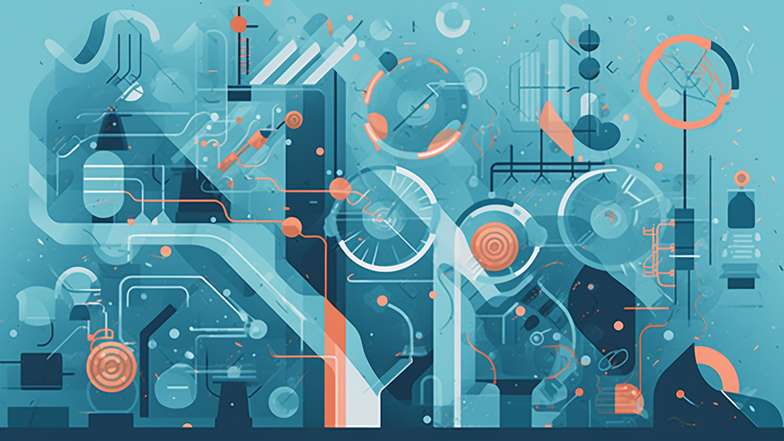
AI technology has the potential to streamline the drug discovery process and improve the efficiency of drug development. By utilizing AI, scientists can analyze vast amounts of data from clinical trials, scientific literature, and other sources, helping them to identify patterns and correlations that might otherwise be missed. In addition to improving the efficiency of the drug discovery process, AI can also help to accurately predict the efficacy and toxicity of new drugs, potentially saving time and resources while also reducing the risks associated with clinical trials.
One of the most exciting applications of AI in drug discovery is its ability to identify new drug targets. With the help of machine learning algorithms, scientists can analyze vast amounts of data to identify potential molecular targets for new drugs. By analyzing large datasets, these algorithms can identify patterns in molecular structures and other characteristics that may correlate with disease, giving researchers valuable insights into potential new targets for drug development.
AI algorithms can also predict how molecules will interact with one another, providing researchers with valuable information about how drugs will behave in the human body. This can help scientists to identify potential safety concerns with new drugs, which can be addressed early in the development process, potentially saving time and resources.
Another important application of AI in drug discovery is in the development of personalized medicine. Traditional drug development focuses on developing drugs that work for the average patient. However, with the help of AI, scientists can analyze patient data to identify subgroups of patients who may be more likely to respond to certain drugs. This can help to improve the efficacy of drugs, while also reducing the risk of adverse side effects.
One example of AI being used to develop personalized medicine is in the treatment of cancer. By analyzing patient data, researchers can identify specific genetic mutations that are associated with certain types of cancer. This information can then be used to develop targeted therapies that are tailored to the individual patient’s genetic profile. This approach has shown promising results, with some targeted therapies showing significant improvements in patient outcomes.
AI can also help to identify new uses for existing drugs. By analyzing large datasets of clinical trial data, scientific literature, and other sources, AI algorithms can identify potential new uses for drugs that were originally developed to treat other conditions. This approach can help to accelerate drug development by identifying new uses for drugs that have already been approved by regulatory agencies, potentially saving time and resources.
However, while AI has the potential to revolutionize the drug discovery process, it is not without its challenges. One of the biggest challenges is the need for large amounts of high-quality data. AI algorithms rely on vast amounts of data to train and improve their accuracy, and the quality of the data can have a significant impact on the accuracy of the predictions made by these algorithms. Generating high-quality data can be a time-consuming and expensive process, which can be a barrier to the adoption of AI in drug discovery.
Another challenge is the need for robust regulatory frameworks. As AI technology becomes more prevalent in drug development processes, regulatory agencies will need to develop frameworks to ensure the safety and efficacy of drugs. This will include developing guidelines for the use of AI in drug development, as well as establishing standards for the evaluation and approval of AI-generated predictions.
In conclusion, the potential of AI to revolutionize the drug discovery process is immense. By analyzing vast amounts of data, AI algorithms can identify potential drug targets, predict drug efficacy and toxicity, and accelerate drug development. However, to fully realize the potential of this technology, researchers will need to overcome a number of challenges, including the need for high-quality data and robust regulatory frameworks. Nevertheless, the promise of personalized medicine and new treatments for diseases gives hope to researchers and patients alike, that with the help of AI, we can improve the way we develop new treatments for diseases and improve healthcare outcomes.
Comments
Post a Comment